In today's world, data annotation plays a crucial role in training machine learning models. Data annotation assessment answers are essential for individuals and organizations looking to improve their annotation skills and ensure high-quality data labeling. If you're diving into this field, understanding these answers can help you succeed in various annotation tasks and evaluations.
Data annotation assessment answers provide valuable insights into the best practices and methodologies for labeling data accurately. By mastering these techniques, professionals can enhance the performance of AI models and contribute to groundbreaking advancements in artificial intelligence.
This article will explore the importance of data annotation, the types of annotation tasks, and the strategies to excel in data annotation assessments. Whether you're a beginner or an experienced annotator, this guide will equip you with the knowledge to tackle any annotation challenge confidently.
Read also:Ultimate Guide To Juan Gabriel Hits A Musical Maestros Legacy
Table of Contents
- Introduction to Data Annotation
- Types of Data Annotation
- Why Data Annotation Assessment Answers Matter
- Strategies for Successful Data Annotation
- Common Challenges in Data Annotation
- Tools for Data Annotation
- Data Annotation Quality Assurance
- Tips for Answering Data Annotation Assessments
- Real-World Applications of Data Annotation
- Future of Data Annotation
Introduction to Data Annotation
Data annotation is the process of labeling or tagging raw data, such as text, images, audio, or video, to make it understandable for machine learning algorithms. This step is critical in the development of AI models because it helps the system recognize patterns and make accurate predictions. High-quality annotations lead to better-performing models, making this task indispensable in the AI industry.
Professionals in this field must understand the nuances of data annotation assessment answers to ensure they meet industry standards. By familiarizing themselves with the various types of annotations and assessment criteria, they can improve their skills and contribute to the development of cutting-edge technologies.
Types of Data Annotation
Text Annotation
Text annotation involves labeling written content for tasks like sentiment analysis, named entity recognition, and part-of-speech tagging. This type of annotation is essential for natural language processing (NLP) models, enabling them to comprehend human language and respond appropriately.
Image Annotation
Image annotation refers to the process of labeling images for computer vision applications. Common techniques include bounding boxes, polygonal segmentation, and keypoint annotation. These annotations help AI models recognize objects, people, and scenes in images.
Audio Annotation
Audio annotation involves labeling sound files for speech recognition and audio classification tasks. This process often includes transcribing spoken words, identifying emotions, and categorizing background noises, making it a vital component of voice-enabled technologies.
Why Data Annotation Assessment Answers Matter
Data annotation assessment answers are crucial for evaluating the quality of annotations and ensuring consistency across projects. By understanding these answers, annotators can identify areas for improvement and refine their skills. Moreover, accurate assessments contribute to the overall success of AI projects, as they guarantee that models are trained on high-quality data.
Read also:Maria Burton Carson A Comprehensive Guide To Her Life Achievements And Legacy
Organizations also benefit from standardized assessments, as they can measure the performance of their annotation teams and allocate resources effectively. This process fosters collaboration and accountability, driving innovation in the AI sector.
Strategies for Successful Data Annotation
Understand the Guidelines
Before starting any annotation task, it's essential to familiarize yourself with the project guidelines and requirements. This step ensures that your annotations align with the intended use case and maintain consistency throughout the dataset.
Utilize Best Practices
- Double-check your work to minimize errors.
- Seek feedback from peers or supervisors to improve your skills.
- Stay updated on the latest trends and techniques in data annotation.
Stay Organized
Efficient organization is key to managing large datasets and meeting deadlines. Use tools and workflows that streamline your annotation process and keep track of your progress.
Common Challenges in Data Annotation
Data annotation is not without its challenges. Some of the most common issues include ambiguous guidelines, inconsistent labeling, and time constraints. To overcome these obstacles, annotators must remain vigilant and proactive in addressing potential problems.
Additionally, the complexity of certain datasets can make annotations more difficult. In such cases, collaboration with domain experts and leveraging advanced tools can help annotators produce high-quality results.
Tools for Data Annotation
Various tools are available to assist annotators in their work, ranging from open-source platforms to proprietary software. Some popular options include:
- Labelbox
- SuperAnnotate
- CVAT
- Amazon SageMaker Ground Truth
These tools offer features like collaborative workflows, quality assurance checks, and integration with machine learning frameworks, making them invaluable assets for data annotation professionals.
Data Annotation Quality Assurance
Importance of Quality Assurance
Quality assurance is vital in data annotation, as it ensures that annotations meet the required standards and are consistent across datasets. Implementing a robust QA process can help organizations reduce errors, improve model performance, and save time and resources.
Methods for Ensuring Quality
- Conduct regular audits of annotated data.
- Implement inter-annotator agreement metrics to measure consistency.
- Use automated tools to detect and correct errors.
Tips for Answering Data Annotation Assessments
When tackling data annotation assessments, consider the following tips to improve your performance:
- Read the instructions carefully and ensure you understand the task requirements.
- Practice regularly to build your skills and confidence.
- Seek feedback from peers or mentors to refine your approach.
By following these guidelines, you can enhance your ability to provide accurate and insightful data annotation assessment answers.
Real-World Applications of Data Annotation
Data annotation plays a pivotal role in numerous real-world applications, including:
Healthcare
In the healthcare sector, data annotation is used for medical imaging analysis, disease diagnosis, and drug discovery. Accurate annotations help improve patient outcomes and streamline healthcare processes.
Autonomous Vehicles
Self-driving cars rely heavily on annotated data to recognize objects, pedestrians, and road conditions. High-quality annotations enable these vehicles to operate safely and efficiently.
Retail
Data annotation is also utilized in retail for tasks like product categorization, customer sentiment analysis, and inventory management. These applications help businesses optimize their operations and enhance customer experiences.
Future of Data Annotation
The future of data annotation looks promising, with advancements in technology and increasing demand for AI-driven solutions. Emerging trends like automation, crowdsourcing, and collaborative annotation platforms are expected to revolutionize the field, making it more accessible and efficient for professionals worldwide.
As the AI industry continues to grow, the importance of data annotation assessment answers will only increase, driving innovation and progress in this exciting domain.
Conclusion
Data annotation assessment answers are a critical component of the AI landscape, enabling professionals to improve their skills and contribute to groundbreaking technologies. By understanding the various types of annotations, strategies for success, and real-world applications, you can excel in this field and make a meaningful impact on the future of artificial intelligence.
We encourage you to share your thoughts and experiences in the comments section below. Additionally, feel free to explore our other articles for more insights into the world of data annotation and AI. Together, we can shape the future of this rapidly evolving industry.
For further reading, check out these reputable sources:
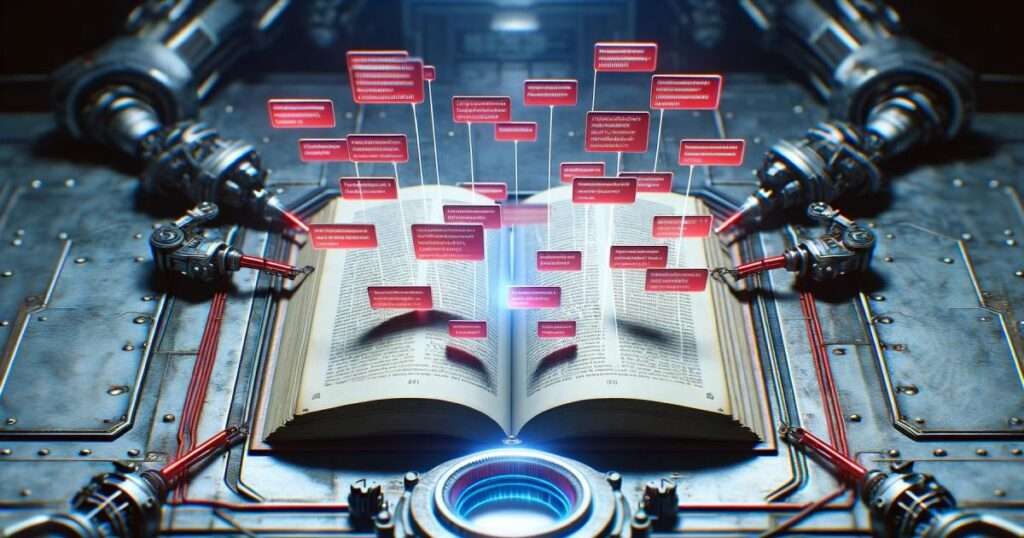
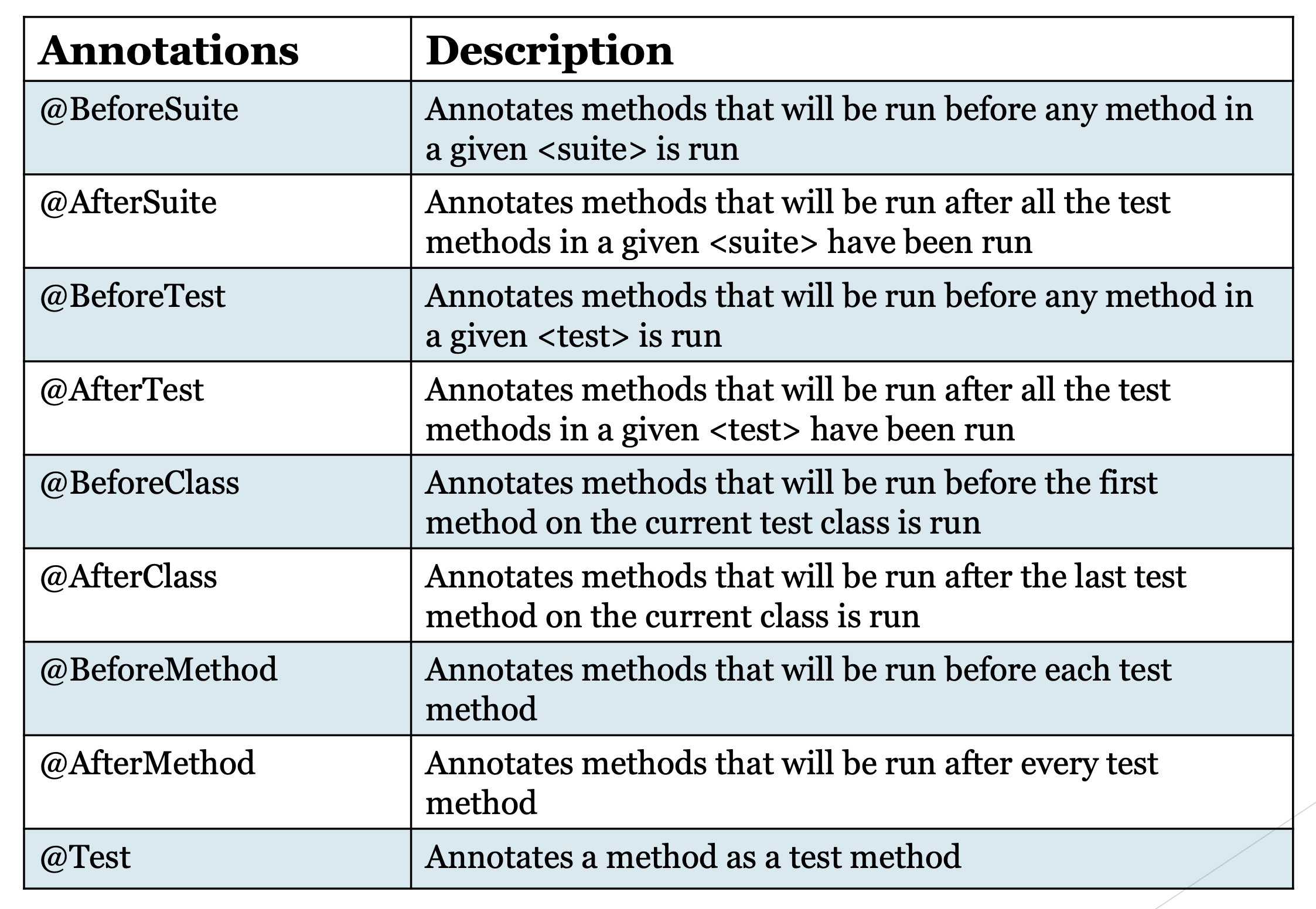